News & Highlights
Topics: In the News, Technology
How Good Are AI ‘Clinicians’ at Medical Conversations?
Researchers design a more realistic test to evaluate AI’s clinical communication skills.
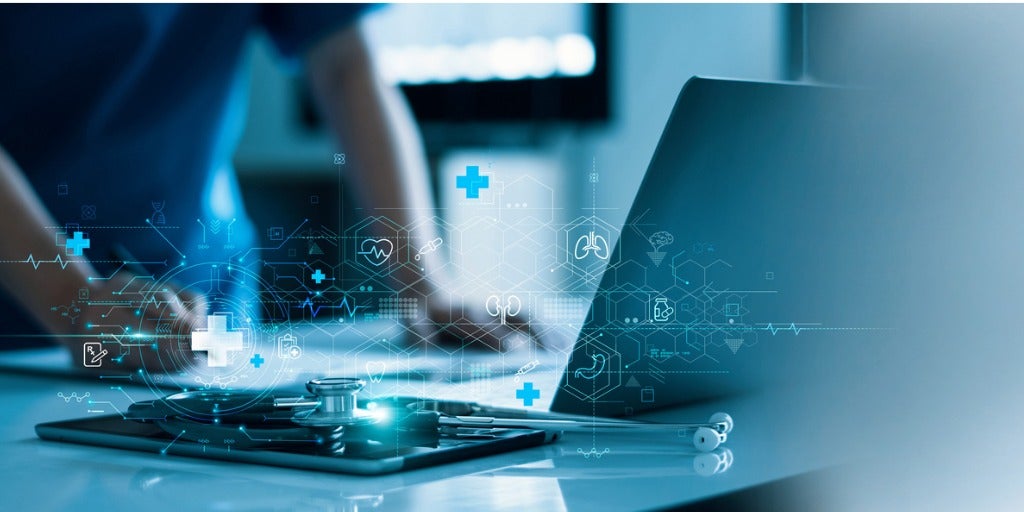
Artificial-intelligence tools such as ChatGPT have been touted for their promise to alleviate clinician workload by triaging patients, taking medical histories, and even providing preliminary diagnoses. These tools, known as large language models, are already being used by patients to make sense of their symptoms and medical test results.
But while these AI models perform impressively on standardized medical tests, how well do they fare in situations that more closely mimic the real world?
Not that great, according to the findings of a new study led by researchers at Harvard Medical School and Stanford University.
For their analysis, published Jan. 2 in Nature Medicine, the researchers designed an evaluation framework—or a test—called CRAFT-MD (Conversational Reasoning Assessment Framework for Testing in Medicine) and deployed it on four large language models to see how well they performed in settings closely mimicking actual interactions with patients.
All four large language models did well on medical exam-style questions, but their performance worsened when engaged in conversations more closely mimicking real-world interactions.
This gap, the researchers said, underscores a two-fold need: First, to create more realistic evaluations that better gauge the fitness of clinical AI models for use in the real world and, second, to improve the ability of these tools to make diagnoses based on more realistic interactions before they are deployed in the clinic.
Evaluation tools like CRAFT-MD, the research team said, can not only assess AI models more accurately for real-world fitness but could also help optimize their performance in the clinic.
“Our work reveals a striking paradox — while these AI models excel at medical board exams, they struggle with the basic back-and-forth of a doctor’s visit.”
“Our work reveals a striking paradox — while these AI models excel at medical board exams, they struggle with the basic back-and-forth of a doctor’s visit,” said study senior author Pranav Rajpurkar, assistant professor of biomedical informatics in the Blavatnik Institute at Harvard Medical School (HMS). “The dynamic nature of medical conversations—the need to ask the right questions at the right time, to piece together scattered information, and to reason through symptoms—poses unique challenges that go far beyond answering multiple-choice questions. When we switch from standardized tests to these natural conversations, even the most sophisticated AI models show significant drops in diagnostic accuracy.”
A better test to check AI’s real-world performance
Right now, developers test the performance of AI models by asking them to answer multiple-choice medical questions, typically derived from the national exam for graduating medical students or from tests given to medical residents as part of their certification.
“This approach assumes that all relevant information is presented clearly and concisely, often with medical terminology or buzzwords that simplify the diagnostic process, but in the real world, this process is far messier,” said study co-first author Shreya Johri, a doctoral student in the Rajpurkar Lab at HMS. “We need a testing framework that reflects reality better and is, therefore, better at predicting how well a model would perform.”
CRAFT-MD was designed to be one such more realistic gauge.
To simulate real-world interactions, CRAFT-MD evaluates how well large language models can collect information about symptoms, medications, and family history and then make a diagnosis. An AI agent is used to pose as a patient, answering questions in a conversational, natural style. Another AI agent grades the accuracy of the final diagnosis rendered by the large language model. Human experts then evaluate the outcomes of each encounter for ability to gather relevant patient information, diagnostic accuracy when presented with scattered information, and adherence to prompts.
The researchers used CRAFT-MD to test four AI models—both proprietary, commercial, and open-source ones—for performance in 2,000 clinical vignettes featuring conditions common in primary care and across 12 medical specialties.
All AI models showed limitations, particularly in their ability to conduct clinical conversations and reason based on information given by patients. That, in turn, compromised their ability to take medical histories and render appropriate diagnoses. For example, the models often struggled to ask the right questions to gather pertinent patient history, missed critical information during history taking, and had difficulty synthesizing scattered information. The accuracy of these models declined when they were presented with open-ended information rather than multiple-choice answers. These models also performed worse when engaged in back-and-forth exchanges—as most real-world conversations are—rather than when engaged in summarized conversations.
Recommendations for optimizing AI’s real-world performance
Based on these findings, the team offers a set of recommendations for both AI developers who design AI models and for regulators charged with evaluating and approving these tools.
These include:
- Use of conversational, open-ended questions that more accurately mirror unstructured doctor-patient interactions in the design, training, and testing of AI tools
- Assessing models for their ability to ask the right questions and to extract the most essential information
- Designing models capable of following multiple conversations and integrating information from them
- Designing AI models capable of integrating textual (notes from conversations) with nontextual data (images, EKGs)
- Designing more sophisticated AI agents that can interpret nonverbal cues such as facial expressions, tone, and body language
Additionally, the researchers recommend that the evaluation should include both AI agents and human experts because relying solely on human experts is labor-intensive and expensive. For example, CRAFT-MD outpaced human evaluators, processing 10,000 conversations in 48 to 72 hours plus 15-16 hours of expert evaluation. In contrast, human-based approaches would require extensive recruitment and an estimated 500 hours for patient simulations (nearly three minutes per conversation) and about 650 hours for expert evaluations (nearly four minutes per conversation). Using AI evaluators as the first line has the added advantage of eliminating the risk of exposing real patients to unverified AI tools.
“CRAFT-MD creates a framework that more closely mirrors real-world interactions and thus it helps move the field forward when it comes to testing AI model performance in healthcare.”
The researchers said they expect that CRAFT-MD itself will also be updated and optimized periodically to integrate improved patient-AI models.
“As a physician-scientist, I am interested in AI models that can augment clinical practice effectively and ethically,” said study co-senior author Roxana Daneshjou, assistant professor of biomedical data science and dermatology at Stanford University. “CRAFT-MD creates a framework that more closely mirrors real-world interactions and thus it helps move the field forward when it comes to testing AI model performance in healthcare.”
Authorship, funding, disclosures
Additional authors include Jaehwan Jeong and Hong-Yu Zhou, Harvard Medical School; Benjamin A. Tran, Georgetown University; Daniel I. Schlessinger, Northwestern University; Shannon Wongvibulsin, University of California-Los Angeles; Leandra A. Barnes, Zhuo Ran Cai, and David Kim, Stanford University; and Eliezer M. Van Allen, Dana-Farber Cancer Institute.
The work was supported by the HMS Dean’s Innovation Award and a Microsoft Accelerate Foundation Models Research grant awarded to Pranav Rajpurkar. Johri received further support through the IIE Quad Fellowship.
Daneshjou reported receiving personal fees from DWA, personal fees from Pfizer, personal fees from L’Oréal, personal fees from VisualDx, stock options from MDAlgorithms and Revea outside the submitted work, and a patent for TrueImage pending. Schlessinger is the co-founder of FixMySkin Healing Balms, a shareholder in Appiell Inc. and K-Health, a consultant with Appiell Inc. and LuminDx, and an investigator for AbbVie and Sanofi. Van Allen serves as an advisor to Enara Bio, Manifold Bio, Monte Rosa, Novartis Institute for Biomedical Research, and Serinus Biosciences and provides research support to Novartis, BMS, Sanofi, and NextPoint. Van Allen holds equity in Tango Therapeutics, Genome Medical, Genomic Life, Enara Bio, Manifold Bio, Microsoft, Monte Rosa, Riva Therapeutics, Serinus Biosciences, and Syapse. Van Allen has filed for institutional patents on chromatin mutations and immunotherapy response and methods for clinical interpretation, provides intermittent legal consulting on patents for Foley Hoag, and serves on the editorial board of Science Advances.