News & Highlights
Topics: Biostatistics, Five Questions
Biostatistics Support for Neuroimaging Researchers Helps Unlock Clinical Potential
Five Questions with biostatistician Lisa Nickerson on advancing translational neuroscience.
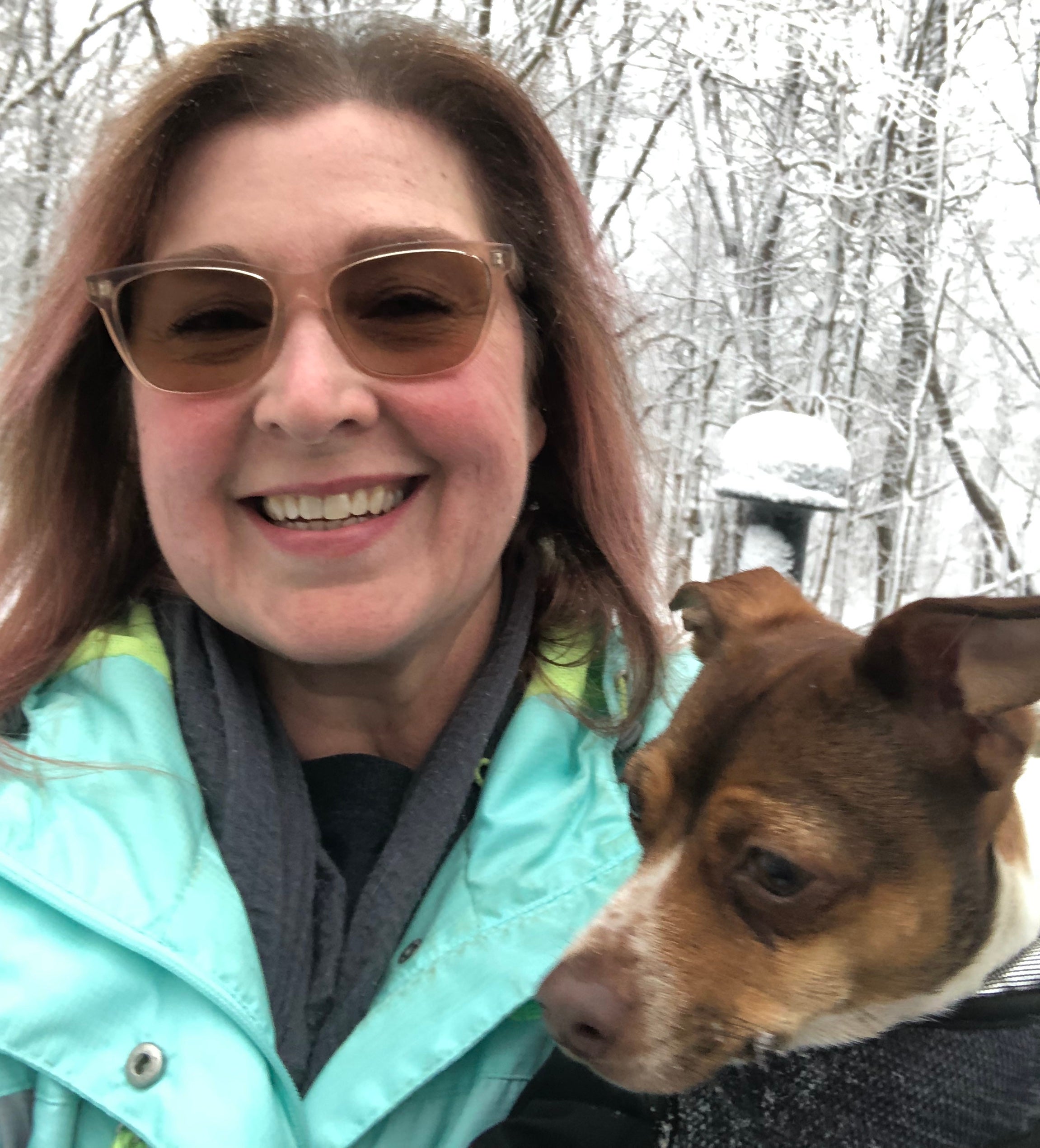
A twelfth-grade physics class changed the course of Lisa Nickerson’s life. Her high school experience had not been ideal, and she had never really thought about college since none of her family members had gone that route. But in that class she found what would become her lifelong calling — physics.
She’s still at it, now as director of the Applied Neuroimaging Statistics Laboratory at McLean Hospital and associate professor of psychiatry at Harvard Medical School. As a postdoc in imaging physics at a time when relevant training programs were rare, she essentially taught herself to analyze functional MRI data, then earned a K grant and headed to Oxford University to train with a world-leading group in neuroimaging analytics.
Nickerson is applying those learnings to unlock the clinical potential of the latest big-data connectome studies in her own newly funded RO1 on Alzheimer’s disease and is helping others do the same with their data as co-investigator on six other NIH-funded grants.
She’s also bringing her skills to a broader array of research as a biostatistician who offers consulting through Harvard Catalyst for Harvard-affiliated researchers at no cost.
As a Catalyst consultant in biostatistics, how do you work with physician-scientists at Harvard and the affiliated hospitals?
Each of the biostatisticians in the program has specific areas of expertise, from clinical trials to machine learning to longitudinal data analysis. Mine is neuroimaging statistics.
“We do countless consultations, helping people from the very beginning of the data analysis stream all the way through to higher-level statistical modeling.”
We do countless consultations, helping people from the very beginning of the data analysis stream all the way through to higher-level statistical modeling. Many are linked to NIH grant deadlines. That’s when people are trying to lay out analysis pipelines to secure funding for their neuroimaging studies. They know what they want to do, but they’re not always sure of the best way to do it, and power calculations required for hypothesis-driven applications in neuroimaging can be quite complicated.
In between grant cycles, requests come from people who want to analyze data they’ve previously collected, or want to do some pilot analyses, or they need an analysis for a paper. They might have reviewers’ comments on a paper and aren’t sure of the best way to address them statistically.
I find that many people feel more comfortable and have more confidence in their grant proposals, study designs, and manuscripts if they have a statistics expert helping them — reviewing their work, giving a seal of approval. I really think it enhances the rigor and reproducibility of research.
People often come for a consultation and realize that having a statistician on the study team strengthens their grant application or manuscript. That’s true for any area of biomedical research, not just neuroimaging.
Any projects you’ve consulted on through Harvard Catalyst that stand out?
Quite a few. One of the more memorable recent grant applications I worked on aims to use neuroimaging to study addiction circuitry in nonhuman primates. I found that really fascinating because there are very different issues involved with animal imaging versus human imaging.
When you’re studying nonhuman primates, your sample size is limited by how many animals you can purchase and care for. So even for a big R01, the sample size is usually really small, 10 to 20 animals. Some would question the value of an N or 10 as not very compelling.
However, in these studies, you are able to control every aspect of that animal’s existence. If you can reduce many of the sources of variability that can contaminate human research, then having an N of 10 or 20 doesn’t seem to be so bad.
Working with the PIs on that grant application was really interesting because I’ve never been involved with a nonhuman primate study. I really hope it gets funded.
Your own research involves applying advanced statistical methods to neuroimaging data to investigate the circuitry of brain disorders across the lifespan. Why this work now?
In neuroimaging, there has been an unfortunate realization that the small sample sizes historically characteristic of imaging studies lead to poor reproducibility of published findings. This limits translation to clinical applications.
Only a couple functional MRI techniques are FDA-approved for clinical use currently. To make progress, particularly in studying brain function and translating fMRI for clinical use, we need to enhance reproducibility and reliability of the technique.
“To make progress, particularly in studying brain function and translating fMRI for clinical use, we need to enhance reproducibility and reliability of the technique.”
The NIH has funded several large-scale neuroimaging studies, including the Human Connectome Project (HCP) lifespan studies and various connectomes related to human disease, comprising about 10,000 participants in total. They’ve also funded the Adolescent Brain & Cognitive Development, a longitudinal study with a data set of 10,000 children across the U.S., to understand how development intersects with addiction and psychiatric disorders that appear in adolescence. And in Europe, the UK Biobank Imaging study is collecting data from 100,000 people.
These big neuroimaging datasets are now all coming online, presenting many opportunities to apply advanced machine-learning techniques that perform best with large sample sizes. I have a great interest in that, which is the focus of my RO1.
How are you tapping into these neuroimaging databanks in your current NIH grant?
The Human Connectome studies span the entire human lifespan from in utero all the way to the oldest old. I’m using data from the HCP young adults study and the HCP aging study, along with several connectomes related to human disease – two for Alzheimer’s and two for neuropsychiatric symptoms such as anxiety and depression.
Altogether this should be enough data and enough diversity of neuropsychiatric symptoms to disentangle these symptoms in Alzheimer’s disease, where they have a different clinical course than in someone who is cognitively normal. Agitation, aggression, anxiety, and depression are incredibly difficult to treat in people with Alzheimer’s and often lead to their institutionalization.
I think mining these big datasets is one way forward for brain imaging, because having these large sample sizes lets you develop normative models. Then you can apply those to smaller boutique studies of special patient populations. You can try to understand disorders that are more difficult to study because you can’t get such large sample sizes. You can do transfer learning, where you train models in big datasets and then use that knowledge in smaller-scale studies.
So there’s great potential both to gain an understanding of brain structure and function in these different populations, and to enhance reproducibility, reliability and understanding of studies across the spectrum.
How do you find work-life balance?
Much of my work involves sitting in front of the computer. As you become more senior and take on more collaborations and responsibilities, you can be locked into computer time a bit more.
Left to my own devices, I will work all day every day, because I love it – but that can lead to health challenges. For me, sitting all day aggravated a hereditary condition and I developed arthritis in my hip joints. So now I have a dog, to make me get outside for walks or weekend excursions. She’s my primary hobby right now. I just love her to pieces.
My other hobby is my 14-year-old great nephew, who has lived with me since he was born. I love doing things with him, which usually involves watching football or taking him to throw the football — all things football-related. We also love to watch the Celtics and had a lot of fun at a recent game.
I help him with his homework too. Next fall, he’ll take physics as a freshman in high school. As a physicist, I’m looking forward to helping him out and it would be my dream if he loved physics as much as I do.