Calendar
Biostatistics Short Course: A Practical Course in Difference-in-Differences Methods – February 13
9:00 am – 1:00 pm
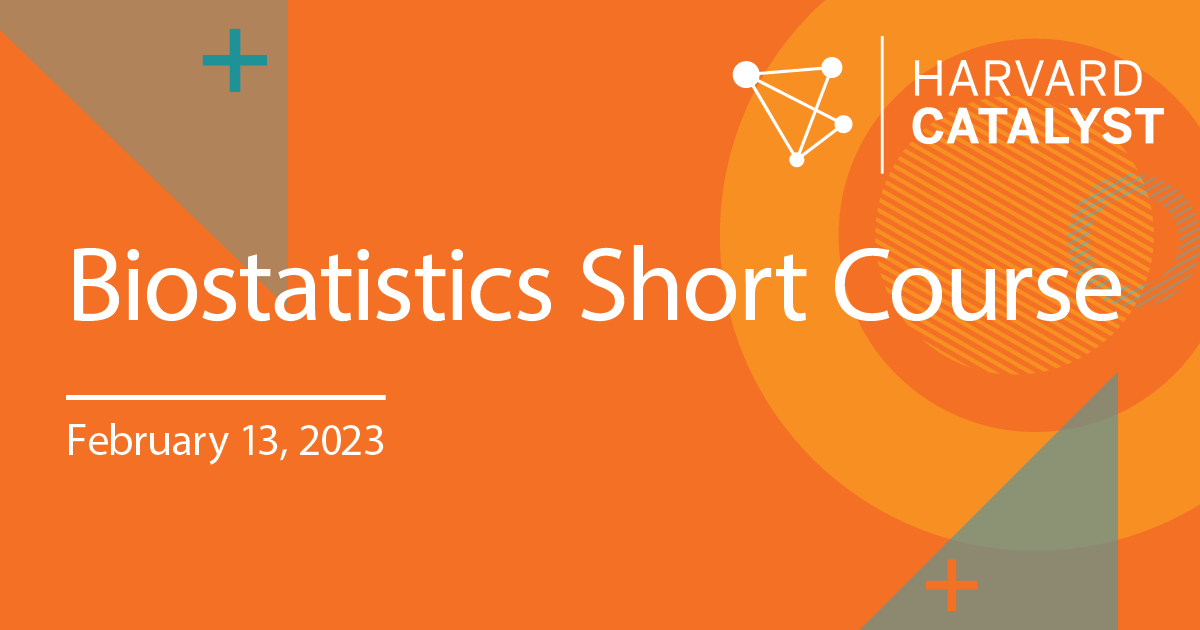
Biostatistics Short Course: A Practical Course in Difference-in-Differences Methods
In this short course, Laura Hatfield, PhD, Harvard Medical School Department of Health Care Policy, will give an in-depth treatment of the fundamentals of causal inference using difference-in-differences methods. This includes formulating target estimands using a potential outcomes framework, applying causal assumptions to identify that estimand, and using basic estimation methods such as regression to estimate the target. We will also cover tests for parallel trends in the pre-intervention period, how to use observed covariates to ameliorate confounding, basic sensitivity analyses, and statistical inference. We will also review alternative identification assumptions, the relationship of difference-in-differences to other methods (like synthetic controls and comparative interrupted time series), and extensions of difference-in-differences to new outcome types. Throughout, we will use real examples from the applied clinical and health policy literatures to illustrate the concepts discussed.
Speaker:
Laura Hatfield, PhD, is an associate professor of health care policy (biostatistics) in the Department of Health Care Policy at Harvard Medical School. Her methods research focuses on causal inference in non-randomized settings, especially using difference-in-differences, and quantifying variation in health care utilization, outcomes, and quality using clustering and hierarchical Bayesian models. Hatfield earned her BS in genetics from Iowa State University and her MS and PhD in biostatistics from the University of Minnesota.
Prerequisites:
This course is intended for researchers who want to be responsible users of difference-in-differences methods but do not have time to keep up with the deluge of new methods developments. Attendees should be familiar with statistics on the level of a year-long graduate-level course in statistical modeling/inference, especially regression-based estimation and inference. Familiarity with basic concepts of causal inference (e.g., potential outcomes, confounding, target estimands, identification assumptions) will also be helpful. The course includes no programming, but the tools recommended to implement the techniques discussed in the course will primarily be in R.