Calendar
Biostatistics Journal Club: Machine Learning for Imputation of Missing-not-at-Random Data – November 15
1:00 pm – 2:00 pm
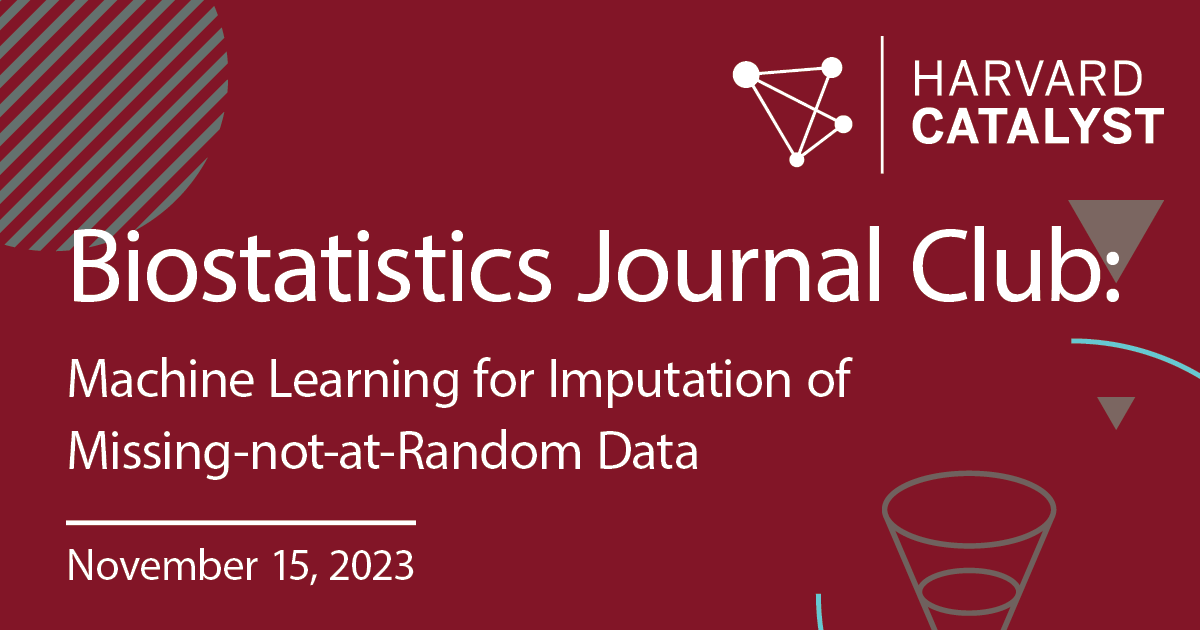
Biostatistics Journal Club: Machine Learning for Imputation of Missing-not-at-Random Data
Missing-not-at-random (MNAR) data frequently appears in real world scenarios, especially when the missing data pattern is also non-monotone. While existing statistical approaches have addressed many well-known MNAR mechanisms, a unified framework for various MNAR assumptions is still lacking. Recently, researchers have started to incorporate modern machine learning techniques into MNAR data analysis to fill in this gap, particularly in methods for missing data imputation. Deep generative networks, with the ability to capture a wide range of underlying data generating mechanisms and to produce new data that closely mimic the observed data, have gained increasing attention as an ideal tool for such purpose. However, many of these machine learning approaches ignore the critical aspect of model identifiability and are thus likely prone to substantial bias. Ma and Zhang attempted to resolve this issue by systematically analyzing the identifiability of generative models under MNAR and propose a practical deep generative model which can provide identifiability guarantees under mild assumptions, for a wide range of MNAR mechanisms. This discussion will first examine the implications of their model assumptions and then explore their link to some of the existing MNAR mechanisms, with the goal to understand the extent to which a deep generative model can serve as a unifying tool for multiple imputation under any generic MNAR mechanism. This discussion will be led by Boyu Ren, PhD, of McLean Hospital.
Speaker:
Boyu Ren, PhD, McLean Hospital
Required Reading:
Identifiable Generative Models for Missing Not at Random Data Imputation